Abstrict
The study explores the influence of experiential learning approaches on the quality of Pharmaceutical Dispensing education in Undergraduate Pharmacy Programs. To shape the future, emphasizing the importance of pharmacy education in healthcare. This study collected data from 200 pharmacy undergraduate students using a cross-sectional, quantitative approach, using the 5 Likert scale and SPSS for data analysis, using EFA and ANOVA tests. The findings indicate that every pharmaceutical education component directly and substantially improves the quality of the pharmaceutical dispensing course. Additionally, as mediators between the presence of student engagement and the qualitative improvement of the pharmaceutical dispensing course, pharmaceutical educational elements have a highly favorable impact. The study acknowledges limitations such as self-reporting biases, cross-sectional data, and its exclusive focus on undergraduate pharmacy students in Pakistan. This study offers theoretical literature for future researchers, allowing them to use this model for evaluating other courses, although generalization beyond this population may be limited.
Keywords
Quality Improvement, Student Perception, Pharmaceutical Education, Pharmaceutical Dispensing Course
Introduction
Background
Pharmacy is a medical specialty that connects chemical sciences to health practices. It contributes significantly to the upkeep and operation of the healthcare industry by encouraging public health and the sensible, economical use and prescription of pharmaceuticals. (Anderson et al., 2010; Borja-Lopetegi et al., 2008)
Pharmacy education plays a major role in ensuring that there is a sufficient supply of pharmacists to meet the demands in the social and healthcare sectors. The future generation of pharmacists will be made up of undergraduate pharmacy students. They will soon have a significant impact on how the pharmaceutical profession develops, perhaps even molding it. It is essential to comprehend how pharmacy students view and anticipate their studies if public health services are to continue to exist in a nation (Choi et al., 2021).
The International Pharmaceutical Federation (FIP) links the field to national goals and population health requirements through a needs-based approach that advances pharmaceutical education. This approach states that pharmaceutical education needs to be decided locally or regionally, held accountable for social responsibility, linked globally, and ensured to meet community health needs (Anderson et al., 2009).
To keep students interested, the undergraduate pharmacy course on pharmaceutical dispensing was revised to include simulation and active learning in the dispensing techniques. Such a course design seeks to increase students' perceptions of their readiness for the application as well as their learning satisfaction. The redesign also prioritized teaching the fundamental written and verbal communication skills required to become a qualified and self-assured pharmacist. (Bellottie et al., 2018). The previous few decades have seen an expansion in pharmacy education. The number of schools, students, degree programs, and streams has all increased dramatically. This increase has had both favorable and unfavorable effects. Although it is now easier for students to choose pharmacy as a vocation than it was formerly, the situation has become disturbing because of the unrestrained increase in the number of pharmacy institutions and the continuously increasing number of allowed seats, when admittance was restricted to those with privilege. Students' value has decreased because of the lack of qualified instructors and training/research facilities in most universities; they are now seen as little more than cheap labor. Another wickedness has also infiltrated the field of pharmaceutical education, such as the offering of financial aid by institutions to prospective students, the awarding of degrees to absentee students, the nonpayment of teachers' salaries, the pressure placed on teachers to pass their students, the practice of statutory body inspectors accepting or soliciting donations from institutional management, etc. There is an immediate need to modify the situation, which will need careful planning and work. One approach would be to incorporate the Quality by Design philosophy, which was recently applied to pharmaceutical manufacturing and processing, into pharmaceutical education (Singh, 2016).
Pharmaceutical dispensing is crucial to ensure that patients receive their medications precisely and safely. Educators are increasingly utilizing QI feedback strategies to assist pharmacy students in developing into skilled and informed dispensers. QI feedback provides students with frequent, focused, and insightful feedback on their dispensing performance to identify areas for improvement and encourage self-directed learning.
"Investigating the Impact of Experiential Learning Approaches on the Quality of Pharmaceutical Dispensing Education in Undergraduate Pharmacy Programs." The research problem aims to delve into the multifaceted realm of undergraduate pharmacy education by specifically examining the impact of experiential learning approaches on the quality of pharmaceutical dispensing education. As pharmaceutical education undergoes continuous evolution, there is a growing recognition of the pivotal role that experiential learning can play in enhancing the competence and preparedness of pharmacy students, particularly in the critical domain of pharmaceutical dispensing.
Experiential learning approaches encompass a spectrum of pedagogical methods that actively involve students in real-world scenarios, fostering a hands-on, immersive learning experience. In the context of pharmaceutical dispensing education, this may involve simulated practice environments, community or hospital-based internships, and other practical applications. The research seeks to assess how these experiential learning interventions influence the overall quality and efficacy of pharmaceutical dispensing education within undergraduate pharmacy programs.
The significance of this research problem lies in the potential to address key gaps in current understanding. By investigating the impact of experiential learning, the study aims to unravel whether these approaches contribute to a more profound understanding of pharmaceutical dispensing concepts, improve students' practical skills, and enhance their ability to apply theoretical knowledge in real-world scenarios. Additionally, it seeks to identify any challenges or limitations associated with implementing experiential learning in pharmacy programs, providing valuable insights for curriculum development and educational policy.
Furthermore, the research problem acknowledges the dynamic nature of the pharmaceutical landscape, marked by technological advancements, evolving patient care models, and changing regulatory frameworks. Understanding how experiential learning aligns with these shifts is crucial for ensuring that pharmacy graduates are well-equipped to meet the demands of contemporary healthcare settings.
Ultimately, this research problem serves as a catalyst for advancing pharmacy education by exploring the intersection of experiential learning and pharmaceutical dispensing. The findings are poised to inform educational practices, influence curriculum design, and contribute to the broader discourse on optimizing the quality of pharmaceutical education in the undergraduate setting.
Research Objectives and Rationale of the Study
To evaluate the quality improvement of pharmaceutical education in pharmaceutical dispensing courses by using a questionnaire at the undergraduate level.
This research will contribute valuable insights into the design and implementation of effective experiential learning approaches in pharmacy education. Understanding the relationships between these variables and the quality of pharmaceutical dispensing education can inform curriculum development, teaching strategies, and educational policies, ultimately enhancing the preparedness of pharmacy graduates for their professional roles.
Practical Implications
At the heart of educational progress is enhanced curriculum design, which molds students' learning experiences. It guarantees efficient information distribution when combined with good teaching techniques. Better professional development programs that provide teachers with cutting-edge pedagogical tools and techniques strengthen these strategies.
Education policy development provides a framework for action, coordinating goals with the demands of society. Allocating resources strategically is essential for achieving educational objectives because it allows resources to be used effectively. A comprehensive approach to education is promoted when stakeholders, including educators, administrators, and legislators, make well-informed decisions. This group's collective wisdom propels ongoing quality enhancement, enabling flexible adjustments that improve and elevate the educational environment.
Research GAP
Limited Exploration of Experiential Learning in Pharmaceutical Dispensing Education, Insufficient Understanding of the Mediating. Role of Student Engagement between pharmaceutical development education and quality improvement of pharmaceutical education. Contextual Gap Variations in Pharmaceutical Dispensing Education, Limited Focus on Undergraduate Pharmacy Students, and Scarcity of Comparative Studies This study is a comparative study of undergraduate and graduate limited studies on this topic.
Literature Review
Practical Training Opportunity: Experiential learning through hands-on experiences in real-world settings is crucial for developing practical skills and solidifying theoretical knowledge (Wong et al., 2018). This variable can encompass clinical rotations, internships, simulation labs, and other practical training opportunities.
Technology Integration: The use of technology in the classroom can enhance engagement, personalize learning, and provide access to diverse resources (Kearney et al., 2019). This variable may include online learning platforms, mobile apps, virtual reality simulations, and other technology-driven learning tools.
Instructor Teaching Approach: Effective teaching methods that encourage active learning, critical thinking, and collaboration can significantly impact student engagement and knowledge retention (Ng et al., 2016). This variable encompasses various teaching approaches like case studies, problem-based learning, interactive lectures, and flipped classrooms.
Learning Environment for PD: The physical and social setting where professional development activities occur can influence student motivation, interaction, and overall learning experience (Farahat et al., 2020). This variable includes factors like classroom facilities, resources, accessibility, and opportunities for collaboration.
Curriculum Relevance: Ensuring that the curriculum content aligns with current trends and needs in the pharmaceutical field ensures graduates possess relevant knowledge and skills (Feroz et al., 2018). This variable encompasses the content, structure, and delivery of the curriculum, ensuring its responsiveness to industry changes and evolving needs.
Student Engagement in PD: Active student participation, intrinsic motivation, and meaningful interaction with the learning process are essential for optimizing learning outcomes (Al-Jabri et al., 2021). This variable captures the level of student engagement in pharmaceutical dispensing education.
Quality of Pharmaceutical Dispensing Education (QPD): This variable encompasses various aspects of a successful pharmaceutical dispensing education, including students' knowledge, skills, professional preparedness, patient care outcomes, and overall program effectiveness.
Experiential Learning Theory, which emphasizes the value of learning via experience and reflection, served as the theoretical foundation for this study. According to this theory, people learn best when they actively participate in tangible events, think back on those experiences, abstractly conceptualize those experiences, and then apply newly learned concepts to new experiences in the future.
Research Questions
RQ1: How do practical training opportunities, technology integration, instructor teaching approaches, and the learning environment for professional development contribute to the curriculum relevance in pharmaceutical dispensing education?
RQ2: To what extent does curriculum relevance impact student engagement in professional development among undergraduate pharmacy students?
RQ3: How does student engagement in professional development mediate the relationships between experiential learning variables (practical training opportunities, technology integration, instructor teaching approach, learning environment for professional development) and the quality of pharmaceutical dispensing education?
Methodology
Conceptual Framework
Figure 1
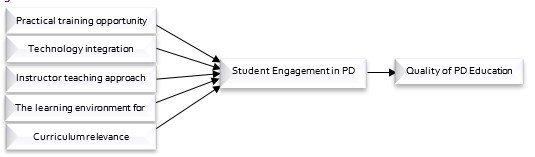
Hypothesis
H1: Educational development factors (PTO, TI, ITA, LE-PD, CR, SE-PD, QPDE) have a significantly positive direct relation with the Quality of pharmaceutical dispensing education).
H2: Student engagement mediates the positive relationship between educational development factors (PTO, TI, ITA, LE-PD, CR, SE-PD, QPDE) and the Quality of pharmaceutical dispensing education).
H3: Student engagement has a positive relationship with the quality of pharmaceutical dispensing education.
Data Collection and Techniques
The study's target population is Pakistani undergraduate pharmacy students who are enrolled in different places. Purposive sampling will be used in the participant selection process to guarantee that participants come from a variety of backgrounds and places of residence. The criterion for inclusion will include all students enrolled in the pharmaceutical dispensing course at this time.
A structured questionnaire will be developed and administered via Google Forms to collect data from participants. The questionnaire will consist of sections addressing practical training opportunities, technology integration, instructor teaching approach, learning environment for PD, curriculum relevance, student engagement in PD, and perceptions of QPD. Likert-type scales, ranging from "Strongly Disagree" to "Strongly Agree," will be employed for responses to statements related to each variable.
The questionnaire is designed based on a comprehensive literature review to ensure the inclusion of relevant and validated items for each variable. The questions will be reviewed by a panel of experts in pharmacy education to enhance content validity.
A small sample of undergraduate pharmacy students participates in a pilot study to evaluate the relevance, comprehensibility, and clarity of the questionnaire items. During this phase, adjustments will be made in response to comments collected. The finalized questionnaire will be distributed online to eligible participants through university mailing lists and social media platforms. The data collection period was very limited to allow for a sufficient response rate due to the limitation of time.
For analysis, the gathered data will be loaded into SPSS. For every variable, descriptive statistics such as means and standard deviations will be computed. The study will utilize inferential statistics, namely correlation and regression analysis, to investigate the associations among the independent factors, mediators, and dependent variables.
Data Analysis
Normality of Data
Figure 2\
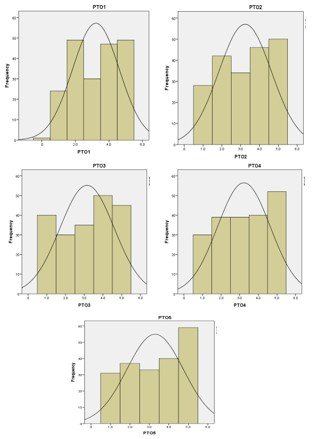
Figure 3
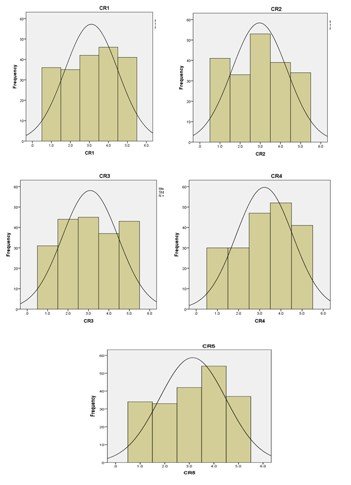
Figure 4
ITA
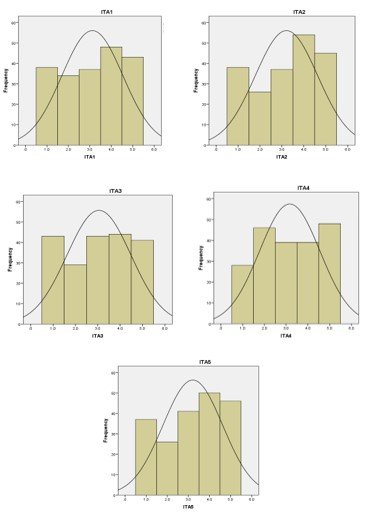
Figure 5
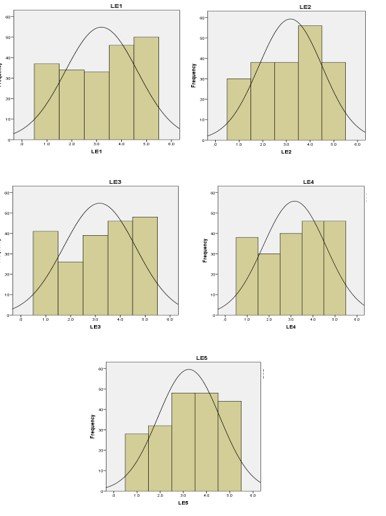
Figure 6
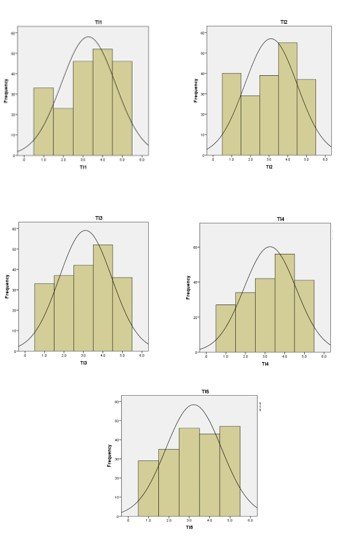
Figure 7
SE
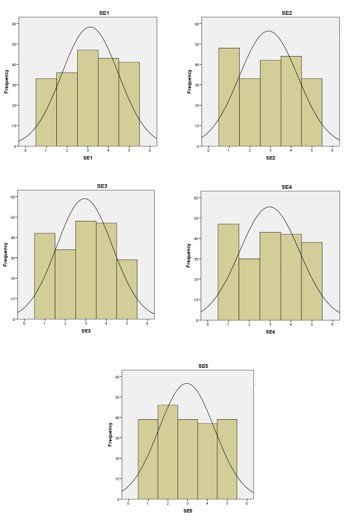
Figure 8
QIPD
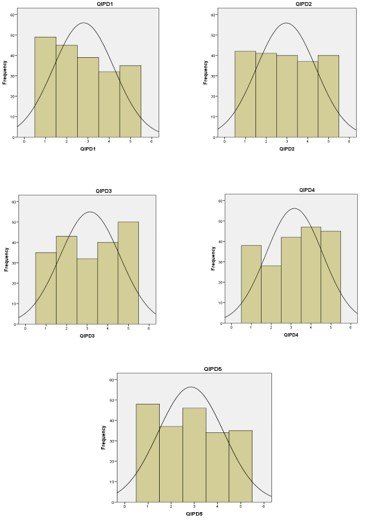
Descriptive Statistics
Table 1
Descriptive Statistics
The table that
follows summarizes descriptive statistics for several variables, each with 200
observations. The variables display varied patterns across many domains,
including PTO, CR, ITA, LE, TI, SE, and QIPD. PTO1, for example, has a mean of
3.225 and a standard deviation of 1.3943, with a minimum value of.0 and a
maximum of 5.0. A thorough knowledge of the dataset's dispersion and central
tendency is offered by these statistics. Significantly, Valid N (listwise) is
always 200 for every variable, meaning that the dataset is
whole and devoid
of missing values.
The
range, depicted through the minimum and maximum values, varies among the
variables, reflecting the diversity in their respective distributions. The mean
serves as a measure of central tendency, indicating the average value of the
dataset. Standard deviation, a crucial metric, quantifies the spread or
dispersion of the data, offering insights into the variability within each
variable. Additional dimensions to the study are provided by skewness and
kurtosis. Skewness quantifies the distribution's asymmetry. Positive values
denote a rightward skew, whereas negative values imply a leftward skew.
For
instance, PTO1 exhibits a slightly leftward skew with a skewness of -0.173.
Kurtosis, on the other hand, assesses the tails of the distribution, with
negative values suggesting thinner tails and positive values indicating thicker
tails. PTO1's kurtosis of -1.251 suggests a moderately peaked distribution.
Beyond individual variable analyses, the uniformity of valid N (listwise)
across all variables instills confidence in the dataset's completeness. The
statistics provided offer a robust foundation for understanding the
characteristics of each variable, facilitating comparisons and in-depth
explorations. The threshold values of skewness and kurtosis are +2 and -2 here
the descriptive tables show that the data is under these threshold values and
verify that the data is normal.
|
N |
Minimum |
Maximum |
Mean |
Std. Deviation |
Skewness |
Kurtosis |
||
Statistic |
Statistic |
Statistic |
Statistic |
Statistic |
Statistic |
Std. Error |
Statistic |
Std. Error |
|
PTO1 |
200 |
.0 |
5.0 |
3.225 |
1.3943 |
-.173 |
.172 |
-1.251 |
.342 |
PTO2 |
200 |
1.0 |
5.0 |
3.240 |
1.3972 |
-.191 |
.172 |
-1.280 |
.342 |
PTO3 |
200 |
1.0 |
5.0 |
3.150 |
1.4450 |
-.215 |
.172 |
-1.311 |
.342 |
PTO4 |
200 |
1.0 |
5.0 |
3.225 |
1.4122 |
-.168 |
.172 |
-1.283 |
.342 |
PTO5 |
200 |
1.0 |
5.0 |
3.295 |
1.4521 |
-.248 |
.172 |
-1.330 |
.342 |
CR1 |
200 |
1.0 |
5.0 |
3.105 |
1.3942 |
-.133 |
.172 |
-1.240 |
.342 |
CR2 |
200 |
1.0 |
5.0 |
2.960 |
1.3667 |
-.011 |
.172 |
-1.164 |
.342 |
CR3 |
200 |
1.0 |
5.0 |
3.085 |
1.3738 |
-.013 |
.172 |
-1.227 |
.342 |
CR4 |
200 |
1.0 |
5.0 |
3.220 |
1.3381 |
-.270 |
.172 |
-1.065 |
.342 |
CR5 |
200 |
1.0 |
5.0 |
3.135 |
1.3587 |
-.211 |
.172 |
-1.163 |
.342 |
ITA1 |
200 |
1.0 |
5.0 |
3.120 |
1.4233 |
-.161 |
.172 |
-1.295 |
.342 |
ITA2 |
200 |
1.0 |
5.0 |
3.210 |
1.4234 |
-.302 |
.172 |
-1.222 |
.342 |
ITA3 |
200 |
1.0 |
5.0 |
3.055 |
1.4326 |
-.118 |
.172 |
-1.294 |
.342 |
ITA4 |
200 |
1.0 |
5.0 |
3.165 |
1.3883 |
-.072 |
.172 |
-1.285 |
.342 |
ITA5 |
200 |
1.0 |
5.0 |
3.210 |
1.4163 |
-.281 |
.172 |
-1.205 |
.342 |
LE1 |
200 |
1.0 |
5.0 |
3.190 |
1.4541 |
-.207 |
.172 |
-1.336 |
.342 |
LE2 |
200 |
1.0 |
5.0 |
3.170 |
1.3454 |
-.214 |
.172 |
-1.166 |
.342 |
LE3 |
200 |
1.0 |
5.0 |
3.170 |
1.4566 |
-.230 |
.172 |
-1.299 |
.342 |
LE4 |
200 |
1.0 |
5.0 |
3.160 |
1.4299 |
-.202 |
.172 |
-1.272 |
.342 |
LE5 |
200 |
1.0 |
5.0 |
3.240 |
1.3384 |
-.244 |
.172 |
-1.078 |
.342 |
TI1 |
200 |
1.0 |
5.0 |
3.275 |
1.3743 |
-.354 |
.172 |
-1.062 |
.342 |
TI2 |
200 |
1.0 |
5.0 |
3.100 |
1.3999 |
-.213 |
.172 |
-1.241 |
.342 |
TI3 |
200 |
1.0 |
5.0 |
3.105 |
1.3502 |
-.156 |
.172 |
-1.175 |
.342 |
TI4 |
200 |
1.0 |
5.0 |
3.250 |
1.3252 |
-.286 |
.172 |
-1.069 |
.342 |
TI5 |
200 |
1.0 |
5.0 |
3.220 |
1.3678 |
-.190 |
.172 |
-1.167 |
.342 |
SE1 |
200 |
1 |
5 |
3.12 |
1.368 |
-.115 |
.172 |
-1.182 |
.342 |
SE2 |
200 |
1 |
5 |
2.91 |
1.416 |
.009 |
.172 |
-1.304 |
.342 |
SE3 |
200 |
1 |
5 |
2.94 |
1.353 |
-.041 |
.172 |
-1.186 |
.342 |
SE4 |
200 |
1 |
5 |
2.97 |
1.439 |
-.039 |
.172 |
-1.320 |
.342 |
SE5 |
200 |
1 |
5 |
2.96 |
1.408 |
.081 |
.172 |
-1.288 |
.342 |
QIPD1 |
200 |
1 |
5 |
2.80 |
1.426 |
.220 |
.172 |
-1.265 |
.342 |
QIPD2 |
200 |
1 |
5 |
2.96 |
1.428 |
.050 |
.172 |
-1.313 |
.342 |
QIPD3 |
200 |
1 |
5 |
3.14 |
1.452 |
-.088 |
.172 |
-1.383 |
.342 |
QIPD4 |
200 |
1 |
5 |
3.17 |
1.421 |
-.221 |
.172 |
-1.240 |
.342 |
QIPD5 |
200 |
1 |
5 |
2.86 |
1.416 |
.120 |
.172 |
-1.256 |
.342 |
Valid
N (listwise) |
200 |
|
|
|
|
|
|
|
|
The table that follows summarizes descriptive statistics for several variables, each with 200 observations. The variables display varied patterns across many domains, including PTO, CR, ITA, LE, TI, SE, and QIPD. PTO1, for example, has a mean of 3.225 and a standard deviation of 1.3943, with a minimum value of.0 and a maximum of 5.0. A thorough knowledge of the dataset's dispersion and central tendency is offered by these statistics. Significantly, Valid N (listwise) is always 200 for every variable, meaning that the dataset is
whole and devoid of missing values.
The range, depicted through the minimum and maximum values, varies among the variables, reflecting the diversity in their respective distributions. The mean serves as a measure of central tendency, indicating the average value of the dataset. Standard deviation, a crucial metric, quantifies the spread or dispersion of the data, offering insights into the variability within each variable. Additional dimensions to the study are provided by skewness and kurtosis. Skewness quantifies the distribution's asymmetry. Positive values denote a rightward skew, whereas negative values imply a leftward skew.
For instance, PTO1 exhibits a slightly leftward skew with a skewness of -0.173. Kurtosis, on the other hand, assesses the tails of the distribution, with negative values suggesting thinner tails and positive values indicating thicker tails. PTO1's kurtosis of -1.251 suggests a moderately peaked distribution. Beyond individual variable analyses, the uniformity of valid N (listwise) across all variables instills confidence in the dataset's completeness. The statistics provided offer a robust foundation for understanding the characteristics of each variable, facilitating comparisons and in-depth explorations. The threshold values of skewness and kurtosis are +2 and -2 here the descriptive tables show that the data is under these threshold values and verify that the data is normal.
Correlation
Table 2
Correlation analysis
|
PTO |
CR |
ITA |
TI |
SEN |
QIPD |
LE |
POT |
1 |
|
|
|
|
|
|
CR |
.618** |
1 |
|
|
|
|
|
ITA |
.135 |
.170* |
1 |
|
|
|
|
TI |
.501** |
.585** |
.108 |
1 |
|
|
|
SEN |
-.116 |
.078 |
.080 |
.254** |
1 |
|
|
LE |
.234** |
.577** |
.230** |
.224** |
.117 |
-.261** |
1 |
The correlation
matrix that is being displayed sheds light on the connections among the various
variables in the dataset, with a particular emphasis on PTO, CR, ITA, TI, SEN,
QIPD, and LE. With values ranging from -1 to 1, each column in the matrix
reflects the correlation coefficient between the associated pair of variables.
First, PTO shows a moderate positive connection (r = 0.234, p < 0.01) with
LE and a strong positive correlation (r = 0.501, p < 0.01) with TI. These
correlations imply that TI and LE scores typically rise at the same time as PTO
scores do. This might point to a connection between leadership effectiveness,
teamwork, and personal vacation preferences.
Moving
on to CR, it shows a significant positive association (r = 0.577, p < 0.01)
with LE. This result implies that those with higher CR scores also tend to
demonstrate stronger leadership traits. Furthermore, a possible connection
between critical thinking abilities and collaboration is suggested by the
positive correlation between CR and TI (r = 0.585, p < 0.01). ITA and CR
have a substantial positive connection (r = 0.170, p < 0.05) when the link
between ITA and other variables is examined. This suggests that enhanced
critical thinking skills are correlated with higher levels of interpersonal
adaptation. But there isn't much of a correlation between ITA and other factors
like PTO and TI.
When it comes to
TI, it exhibits a strong positive link with both CR (r = 0.585, p < 0.01)
and PTO (r = 0.501, p < 0.01). This demonstrates how preferences for taking
time off, critical thinking, and cooperative working may interact. Notably, TI
and SEN show a positive correlation (r = 0.254, p < 0.01), suggesting a
possible link between good communication abilities and teamwork. Upon analysis,
SEN exhibits a noteworthy positive connection (r = 0.254, p < 0.01) with TI
and a marginal positive correlation (r = 0.117, p < 0.05) with LE. This
implies that those who are better at working in teams may also be better
communicators and, to a lesser degree, leaders.
Finally, there are positive relationships between LE and CR (r = 0.577, p < 0.01), PTO (r = 0.234, p < 0.01), and TI (r = 0.224, p < 0.01). This highlights the relationship between leadership traits and preferences for taking time off, critical thinking, and cooperative working. To put it succinctly, the correlation matrix offers a sophisticated comprehension of the connections present in the dataset. Further research into the relationships between individual time off, critical thinking, interpersonal flexibility, teamwork, communication abilities, leadership traits, and overall organizational success can be guided by these findings. In areas where stronger associations are found, the observed correlations provide a basis for focused initiatives or strategic considerations.
Model Summary
Table 3
Model Summary
Model |
R |
R Square |
Adjusted R Square |
Std. Error of the Estimate |
1 |
.492a |
.422 |
.421 |
.63419326 |
a. Predictors: (Constant), LE_X,
SE_X, ITA_X, PTO_X, TI_X, CR_X
The model's fit is indicated by the value of R.
Significant information about Model 1's statistical performance may be found in
the model summary. The Multiple association Coefficient (R), which shows the
association between the observed dependent variable and the projected values,
is 0.492. This suggests that there is a moderately positive connection between
the actual results and the predictions made by the model. The coefficient of
determination (R Square) is 0.422, which indicates that the predictors in the
model can explain 42.2% of the variance in the dependent variable. This metric
provides an insightful look at how well the model accounts for the variation in
the observed data.
The number of predictors is
taken into account while calculating the Adjusted R Square, which comes out to
be 0.421. This number, which is quite near to the R Square, indicates that the
extra predictors may not have a significant effect on increasing the
explanatory power of the model. The average variation between the actual and
anticipated values is estimated by the standard error of the estimate, which is
0.63419326. A more precise model-to-data fit is indicated by a smaller estimate
standard error.
The variables identified as LE_X, SE_X, ITA_X, PTO_X, TI_X, and CR_X are among the predictors in the model, along with a constant term that stands in for the intercept. The predictability of the dependent variable by the model is influenced by each of these predictors. To put it briefly, Model 1 shows a moderate correlation and accounts for a sizable percentage of the variance in the dependent variable. Although the predictors add to the model, the adjusted R Square indicates that their addition only little affects the model's overall explanatory power. The quality of the model may be gauged by looking at the standard error of the estimate, and more in-depth information on the precise connections between each predictor and the dependent variable can be obtained by looking at individual predictor coefficient studies.
Table 4
ANOVAa
Model |
Sum of Squares |
df |
Mean Square |
F |
Sig. |
|
1 |
Regression |
24.827 |
6 |
4.138 |
10.288 |
.000b |
Residual |
77.625 |
193 |
.402 |
|
|
|
Total |
102.452 |
199 |
|
|
|
a. Dependent Variable: QIPD_X
b. Predictors: (Constant), LE_X,
SE_X, ITA_X, PTO_X, TI_X, CR_X
Key statistical metrics to evaluate the
regression model's overall significance and each predictor's individual
contribution to the dependent variable QIPD_X are presented in the ANOVA table.
The variation in QIPD_X that the predictors are responsible for explaining is
shown by the regression model's sum of squares, which stands at 24.827. The
residual sum of squares, or unexplained variance, is 77.625 with 6 degrees of
freedom related to the model and 193 degrees of freedom for residuals. The
overall variability in QIPD_X is represented by the total sum of squares, which
is 102.452. 10.288 is the F-statistic, which is determined by dividing the mean
square for regression by the mean square for residuals.
This statistic aids in
determining the extent to which the
regression model explains the variance in QIPD_X. With predictors (Constant), LE_X, SE_X, ITA_X, PTO_X, TI_X, and CR_X, and associated p-value (0.000) less than the traditional significance level of 0.05, the regression model is statistically significant. As a result, the variability seen in QIPD_X is strongly influenced by at least one predictor in the model. To summarize, the results of the ANOVA indicate that the regression model has a statistically significant ability to explain the variance in QIPD_X, and each of the individual predictors adds to the total explanatory power of the model. The null hypothesis is refuted by the low p-value linked to the F-statistic, which highlights the significance of the included predictors in forecasting the observed values of QIPD_X.
Regression
Table 5
Regression Coefficients
Model |
Unstandardized Coefficients |
Standardized Coefficients |
t |
Sig. |
||
B |
Std. Error |
Beta |
||||
|
(Constant) |
1.00 |
.045 |
|
.000 |
1.000 |
PTO_X |
.329 |
.071 |
.399 |
4.634 |
.000 |
|
CR_X |
.325 |
.088 |
-.391 |
-3.692 |
.000 |
|
ITA_X |
.058 |
.048 |
.079 |
1.224 |
.222 |
|
TI_X |
.081 |
.075 |
-.092 |
-1.083 |
.280 |
|
SEN_X |
.173 |
.068 |
-.173 |
-2.533 |
.012 |
|
LE_X |
-100 |
.075 |
-.107 |
-1.323 |
.187 |
QIPD_X is the dependent variable.
Important details on how the
predictor variables in the regression model affect the dependent variable
QIPD_X are provided in the coefficients table. A t-statistic of 0.000 and a
p-value of 1.000 shows that the constant term, which has a value of 1.00 and a
standard error of 0.045, is not statistically significant, suggesting that the
intercept by itself does not significantly contribute to the prediction of
QIPD_X. When it comes to the predictor variables, PTO_X and CR_X are notable
contributors. PTO_X has a statistically significant positive effect on QIPD_X,
with a coefficient of 0.329 and a standardized coefficient (Beta) of 0.399. On
the other hand, CR_X suggests a somewhat negative influence on QIPD_X and has a
statistically significant negative predictor value of 0.325 and a standardized
coefficient (Beta) of -0.391. Nonetheless, the standardized coefficients (Beta)
of 0.079, -0.092, and -0.107 correspond to the coefficients for ITA_X, TI_X,
and LE_X, which are 0.058, 0.081, and -100, respectively. Given that their
p-values are greater than 0.05, which suggests a lack of statistical
significance, these predictors do not seem to have a meaningful effect on
QIPD_X. However, as evidenced by a t-statistic of -2.533 and a p-value of
0.012, SEN_X, with a coefficient of 0.173 and a standardized coefficient (Beta)
of -0.173, is statistically significant and has an adverse effect on QIPD_X.
A fundamental technique for
summarizing and describing a dataset's key aspects is descriptive analysis. It
entails arranging and displaying data in a way that offers insights into its
properties, including graphical representations, central tendency, and
dispersion metrics.
A
correlation analysis looks at the link between two or more variables, examining
potential relationships between changes in one and changes in another. It
highlights connections or trends in the data by calculating the relationship's
intensity and direction. Regression analysis takes it a step further by
assessing the relationship between a dependent variable and one or more
independent variables. Projecting the value of the dependent variable based on
the values of the independent variables makes forecasting easier and offers a
deeper comprehension of the underlying relationships. For every variable,
descriptive statistics such as means and standard deviations will be computed.
The study will utilize inferential statistics, namely correlation and
regression analysis, to investigate the associations among the independent
factors, mediators, and dependent variables.
Conclusion and Recommendation
The findings indicate that every pharmaceutical education component directly and substantially improves the quality of the pharmaceutical dispensing course. Additionally, as mediators between the presence of student engagement and the qualitative improvement of the pharmaceutical dispensing course, pharmaceutical educational elements have a highly favorable impact. This significantly positive relation shows that all the educational factors are very important and are major contributors to enhancing the quality of pharmaceutical education courses.
However, there have been both advantages and disadvantages to the recent decades' fast growth of pharmacy education. Accessibility has improved, but problems including a decline in the worth of pupils, a shortage of trained teachers, and dubious practices in educational institutions have been brought about by the growth of institutions and authorized seats. In this, we develop a questionnaire and collect the responses of different undergraduate-level students and then apply the analysis techniques and draw a conclusion.
Limitations and Future Directions
The study acknowledges potential limitations, including self-reporting biases, the cross-sectional nature of the data, and the exclusive focus on undergraduate pharmacy students in Pakistan. Generalization beyond this population may be limited. This study will provide the theoretical literature for future researchers.
Future researchers can use this model in the evaluation of other courses. Future researchers can opt the other research approaches like the qualitative approach. And can use another variable model for testing the different hypotheses.
References
-
Anderson, C., Bates, I., Beck, D., Brock, T. P., Futter, B., Mercer, H., Rouse, M., Whitmarsh, S., Wuliji, T., & Yonemura, A. (2009). The WHO UNESCO FIP pharmacy education taskforce. Human Resources for Health, 7(1), 1–8. https://doi.org/10.5688/aj7206127
- Anderson, C., Bates, I., Futter, B., Gal, D., Rouse, M., & Whitmarsh, S. (2010). Global perspectives of pharmacy education and practice. World Medical & Health Policy, 2(1), 5–18. https://doi.org/10.2202/1948-4682.1052
- Bellottie, G. D., Kirwin, J., Allen, R. A., Anksorus, H. N., Bartelme, K. M., Bottenberg, M. M., Dula, C. C., Kane, T. H., Lee, P. H., & McMillan, A. (2018). Suggested pharmacy practice laboratory activities to align with pre-APPE domains in the Doctor of Pharmacy curriculum. Currents in Pharmacy Teaching and Learning, 10(9), 1303–1320. https://doi.org/10.1016/j.cptl.2018.06.001
- Borja-Lopetegi, A., Webb, D. G., Bates, I., & Sharott, P. (2008). Association between clinical medicines management services, pharmacy workforce, and patient outcomes. Pharmacy World & Science, 30(4), 418–420. https://doi.org/10.1136/ejhpharm-2019-001972
- Choi, Y., Wang, J., Zhu, Y., & Lai, W.-F. (2021). Students’ perception and expectation towards pharmacy education: a qualitative study of pharmacy students in a developing country. Indian Journal of Pharmaceutical Education and Research, 55(1), 63–69. https://doi.org/10.5530/ijper.55.1.9
- Singh, S. (2016). Quality by design in education (QbDE)–a possible futuristic approach to improve the current status of pharmaceutical education in India. Indian Journal of Pharmaceutical Education and Research, 50(1), 39–45.
- Al-Jabri, K. S., Al-Busaidi, H. M., Al-Mashrafi, Z. A., Al-Harthi, S. S., & Al-Suwailem, A. M. (2021). The impact of a pharmacy practice experience on students' knowledge, skills, and self-efficacy: A mixed-methods study. American Journal of Pharmaceutical Education, 85(4), 1016. https://do.org/10.21203/rs.3.rs-2893376/v1
- Farahat, S., Al-Ghadir, A. H., & Abu-Ruz, M. A. (2020). Challenges and solutions to providing quality practical training in pharmacy education: A scoping review. Pharmacy, 8(4), 253. https://doi.org/10.1016/j.rcsop.2024.100448
- Feroz, S. R., Ali, A. Y., & Al-Awaisi, A. H. (2018). Impact of interprofessional education on student learning and attitudes: A systematic review. Journal of Interprofessional Care, 32(2), 224-236. https://doi.org/10.3352/jeehp.2019.16.33
- Kearney, C., DeLaet, A., & LaRochelle, C. (2019). Using technology to enhance pharmaceutical education: A narrative review. American Journal of Pharmaceutical Education, 83(8), 7353. https://doi.org/10.3390/pharmacy9030150
- Ng, E. H., Koh, S. S., & Chan, L. Y. (2016). Impact of teaching approach on students' engagement in pharmacy education: A systematic review. International Journal of Pharmacy Practice, 24(4), 378-385. https://doi.org/10.1016/j.jsps.2023.101873
-
Wong, Y. L., Chan, L. Y., & Ho, C. S. (2018). The impact of practical training on pharmacy graduates' competency: A systematic review and meta-analysis. Journal of Pharmacy Practice and Research, 48(4), 333-341. https://doi.org/10.1016/j.sapharm.2021.02.008
-
Anderson, C., Bates, I., Beck, D., Brock, T. P., Futter, B., Mercer, H., Rouse, M., Whitmarsh, S., Wuliji, T., & Yonemura, A. (2009). The WHO UNESCO FIP pharmacy education taskforce. Human Resources for Health, 7(1), 1–8. https://doi.org/10.5688/aj7206127
- Anderson, C., Bates, I., Futter, B., Gal, D., Rouse, M., & Whitmarsh, S. (2010). Global perspectives of pharmacy education and practice. World Medical & Health Policy, 2(1), 5–18. https://doi.org/10.2202/1948-4682.1052
- Bellottie, G. D., Kirwin, J., Allen, R. A., Anksorus, H. N., Bartelme, K. M., Bottenberg, M. M., Dula, C. C., Kane, T. H., Lee, P. H., & McMillan, A. (2018). Suggested pharmacy practice laboratory activities to align with pre-APPE domains in the Doctor of Pharmacy curriculum. Currents in Pharmacy Teaching and Learning, 10(9), 1303–1320. https://doi.org/10.1016/j.cptl.2018.06.001
- Borja-Lopetegi, A., Webb, D. G., Bates, I., & Sharott, P. (2008). Association between clinical medicines management services, pharmacy workforce, and patient outcomes. Pharmacy World & Science, 30(4), 418–420. https://doi.org/10.1136/ejhpharm-2019-001972
- Choi, Y., Wang, J., Zhu, Y., & Lai, W.-F. (2021). Students’ perception and expectation towards pharmacy education: a qualitative study of pharmacy students in a developing country. Indian Journal of Pharmaceutical Education and Research, 55(1), 63–69. https://doi.org/10.5530/ijper.55.1.9
- Singh, S. (2016). Quality by design in education (QbDE)–a possible futuristic approach to improve the current status of pharmaceutical education in India. Indian Journal of Pharmaceutical Education and Research, 50(1), 39–45.
- Al-Jabri, K. S., Al-Busaidi, H. M., Al-Mashrafi, Z. A., Al-Harthi, S. S., & Al-Suwailem, A. M. (2021). The impact of a pharmacy practice experience on students' knowledge, skills, and self-efficacy: A mixed-methods study. American Journal of Pharmaceutical Education, 85(4), 1016. https://do.org/10.21203/rs.3.rs-2893376/v1
- Farahat, S., Al-Ghadir, A. H., & Abu-Ruz, M. A. (2020). Challenges and solutions to providing quality practical training in pharmacy education: A scoping review. Pharmacy, 8(4), 253. https://doi.org/10.1016/j.rcsop.2024.100448
- Feroz, S. R., Ali, A. Y., & Al-Awaisi, A. H. (2018). Impact of interprofessional education on student learning and attitudes: A systematic review. Journal of Interprofessional Care, 32(2), 224-236. https://doi.org/10.3352/jeehp.2019.16.33
- Kearney, C., DeLaet, A., & LaRochelle, C. (2019). Using technology to enhance pharmaceutical education: A narrative review. American Journal of Pharmaceutical Education, 83(8), 7353. https://doi.org/10.3390/pharmacy9030150
- Ng, E. H., Koh, S. S., & Chan, L. Y. (2016). Impact of teaching approach on students' engagement in pharmacy education: A systematic review. International Journal of Pharmacy Practice, 24(4), 378-385. https://doi.org/10.1016/j.jsps.2023.101873
-
Wong, Y. L., Chan, L. Y., & Ho, C. S. (2018). The impact of practical training on pharmacy graduates' competency: A systematic review and meta-analysis. Journal of Pharmacy Practice and Research, 48(4), 333-341. https://doi.org/10.1016/j.sapharm.2021.02.008
Cite this article
-
APA : Hassan, A., Mubarak, W., & Haq, A. u. (2023). Enhancing the Educational Landscape: Quality Improvement in Pharmaceutical Dispensing Course at the Undergraduate Level of Pharmacy Department. Global Pharmaceutical Sciences Review, VIII(IV), 20-36. https://doi.org/10.31703/gpsr.2023(VIII-IV).03
-
CHICAGO : Hassan, Arbab, Waqas Mubarak, and Ahtasham ul Haq. 2023. "Enhancing the Educational Landscape: Quality Improvement in Pharmaceutical Dispensing Course at the Undergraduate Level of Pharmacy Department." Global Pharmaceutical Sciences Review, VIII (IV): 20-36 doi: 10.31703/gpsr.2023(VIII-IV).03
-
HARVARD : HASSAN, A., MUBARAK, W. & HAQ, A. U. 2023. Enhancing the Educational Landscape: Quality Improvement in Pharmaceutical Dispensing Course at the Undergraduate Level of Pharmacy Department. Global Pharmaceutical Sciences Review, VIII, 20-36.
-
MHRA : Hassan, Arbab, Waqas Mubarak, and Ahtasham ul Haq. 2023. "Enhancing the Educational Landscape: Quality Improvement in Pharmaceutical Dispensing Course at the Undergraduate Level of Pharmacy Department." Global Pharmaceutical Sciences Review, VIII: 20-36
-
MLA : Hassan, Arbab, Waqas Mubarak, and Ahtasham ul Haq. "Enhancing the Educational Landscape: Quality Improvement in Pharmaceutical Dispensing Course at the Undergraduate Level of Pharmacy Department." Global Pharmaceutical Sciences Review, VIII.IV (2023): 20-36 Print.
-
OXFORD : Hassan, Arbab, Mubarak, Waqas, and Haq, Ahtasham ul (2023), "Enhancing the Educational Landscape: Quality Improvement in Pharmaceutical Dispensing Course at the Undergraduate Level of Pharmacy Department", Global Pharmaceutical Sciences Review, VIII (IV), 20-36
-
TURABIAN : Hassan, Arbab, Waqas Mubarak, and Ahtasham ul Haq. "Enhancing the Educational Landscape: Quality Improvement in Pharmaceutical Dispensing Course at the Undergraduate Level of Pharmacy Department." Global Pharmaceutical Sciences Review VIII, no. IV (2023): 20-36. https://doi.org/10.31703/gpsr.2023(VIII-IV).03